The Ultimate Guide to Image Labeling for Businesses
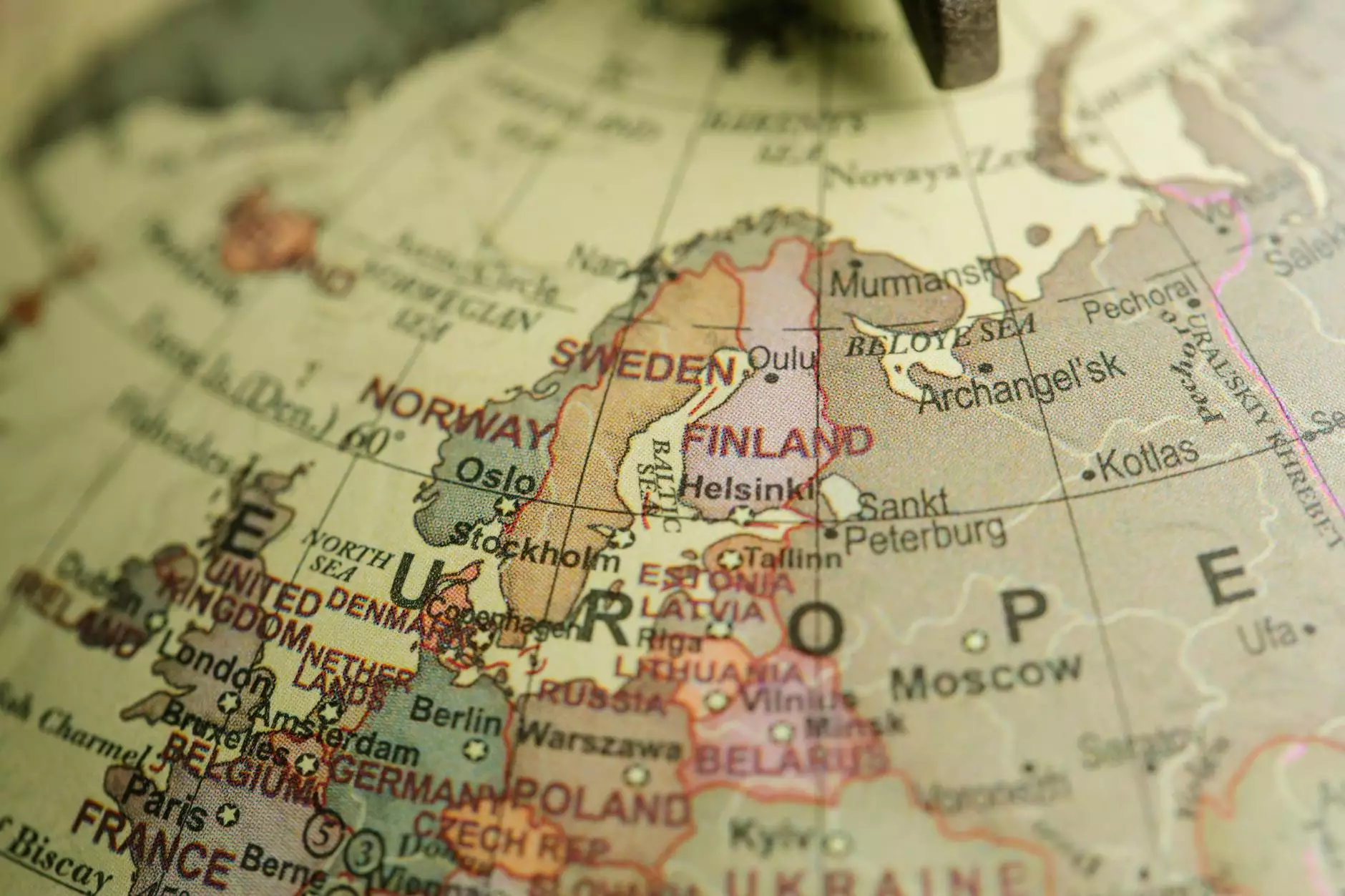
Image labeling has become a cornerstone of modern data annotation practices, crucial for businesses that rely on artificial intelligence (AI) and machine learning (ML). The significance of this process extends beyond mere labeling—it serves as the bedrock for developing highly effective models that derive insights from vast datasets. In this comprehensive guide, we will explore the various aspects of image labeling, its relevance in today’s business landscape, and how platforms like KeyLabs.ai are revolutionizing data annotation tools and methodologies.
What is Image Labeling?
Image labeling involves the process of identifying and tagging specific objects within images. This practice is critical in training AI systems to recognize various entities, enabling functionalities such as object detection, image classification, and segmentation. Labeling can range from simple tasks—such as identifying a single object in a picture—to complex labeling, where multiple items or activities must be annotated within a single frame.
Why is Image Labeling Essential for Businesses?
In the digital age, the ability to derive actionable insights from unstructured data is paramount. Here are just a few reasons why image labeling holds such importance:
- Facilitates Machine Learning: Quality labeled images are essential for creating training datasets that empower machine learning algorithms to function effectively.
- Improves Product Recognition: Businesses can enhance their product visibility and recognition within e-commerce platforms by ensuring their product images are accurately labeled.
- Boosts User Experience: Image labeling contributes to better search functionality in apps and websites, providing users with more intuitive navigation.
- Supports Automated Processes: Accurate image labeling supports automation in industries like logistics, healthcare, and automotive, streamlining operations and reducing costs.
How Does Image Labeling Work?
The process of image labeling typically follows several critical steps:
- Data Collection: Gather a comprehensive dataset containing images relevant to your business.
- Annotation Tool Selection: Choose a robust data annotation tool that meets your needs—like KeyLabs.ai—to facilitate the labeling process.
- Label Definition: Define the categories and attributes you want to label within your images.
- Manual or Automated Annotation: Depending on your tool, choose whether annotations will be performed manually by a human or automatically by an AI system.
- Quality Assurance: Implement a thorough review process to ensure labeled data accuracy and consistency.
- Integration: Incorporate the labeled data into your machine learning models for training.
The Role of KeyLabs.ai in Image Labeling
KeyLabs.ai provides an innovative platform for image labeling tailored to meet the needs of diverse industries. Here are some stellar features of the KeyLabs.ai annotation platform:
1. User-Friendly Interface
The platform is designed with usability in mind, allowing users to easily navigate through the image labeling process without extensive technical knowledge.
2. Versatility Across Industries
Whether you're in healthcare, automotive, retail, or any other sector, KeyLabs.ai accommodates various image labeling needs tailored to your industry requirements.
3. High Accuracy Rates
By leveraging advanced algorithms and human oversight, KeyLabs.ai ensures that your labeled data achieves a high degree of accuracy—essential for successful AI training.
4. Scalability
As your business grows, so does your need for labeled data. The platform can handle extensive datasets effortlessly, making it suitable for startups and established enterprises alike.
5. Collaboration Features
Multiple team members can work on the same project concurrently, enabling real-time collaboration to enhance productivity and efficiency.
Types of Image Labeling Techniques
Understanding different labeling techniques is critical for businesses to tailor their approaches effectively. Here are some commonly used techniques:
1. Semantic Segmentation
This technique involves classifying each pixel of an image into designated categories. It is particularly useful in applications such as autonomous vehicles where understanding the environment is paramount.
2. Bounding Boxes
Bounding boxes are the most common form of image labeling. They involve drawing rectangles around objects of interest. This method is widely used in object detection tasks.
3. Polygon Annotation
For more complex shapes, polygon annotation allows for precise outlining of an object. This technique is crucial when dealing with irregularly shaped items.
4. Landmark Annotation
In this approach, specific points of interest on an object are marked. This is particularly useful in facial recognition and medical imaging.
5. Line and Path Annotation
This technique is used to annotate edges and lines, which is especially useful in satellite imagery and mapping applications.
Best Practices for Image Labeling
To ensure high-quality output, consider these best practices when performing image labeling tasks:
- Define Clear Guidelines: Establish comprehensive labeling guidelines for consistency across all annotators.
- Training for Annotators: Invest in training sessions for team members involved in the labeling process to improve quality and consistency.
- Automate Where Possible: Utilize AI-driven tools to initiate the labeling process and reduce manual workloads.
- Regular Quality Checks: Implement a system of regular review and quality checks to maintain high standards.
- Feedback Loop: Create a feedback mechanism for annotators to learn from errors and improve over time.
Future Trends in Image Labeling
The landscape of image labeling continues to evolve with advancements in AI and machine learning technologies. Here are some trends to watch for:
1. Enhanced Automation
As machine learning models become more sophisticated, automation in labeling processes will increase, reducing the time and effort required for manual annotation.
2. Integration with Augmented Reality (AR)
AR technologies will leverage image labeling to enrich user experiences by providing real-time information and interactions with physical spaces.
3. Crowdsourced Solutions
Crowdsourcing will play a more significant role in image labeling, providing diverse perspectives and accelerating the annotation process through community contributions.
4. Ethical Considerations
As businesses utilize image labeling more widely, the ethical implications of data usage and AI deployment will demand greater scrutiny and accountability.
Conclusion
In conclusion, image labeling is more than just a technical necessity; it's a crucial aspect of business that can significantly impact operational efficiency and the overall success of AI-driven initiatives. Platforms like KeyLabs.ai offer comprehensive solutions tailored for high-quality data annotations, ensuring that your projects succeed in leveraging the potential of labeled images.
Investing time and resources into effective image labeling strategies can yield substantial returns, making it an invaluable practice for any forward-thinking organization aiming to thrive in a data-driven world. As AI and machine learning continue to evolve, embracing the best practices and leveraging robust platforms will empower businesses to harness the true power of their data.