Enhancing Machine Learning with Video Annotation Tools
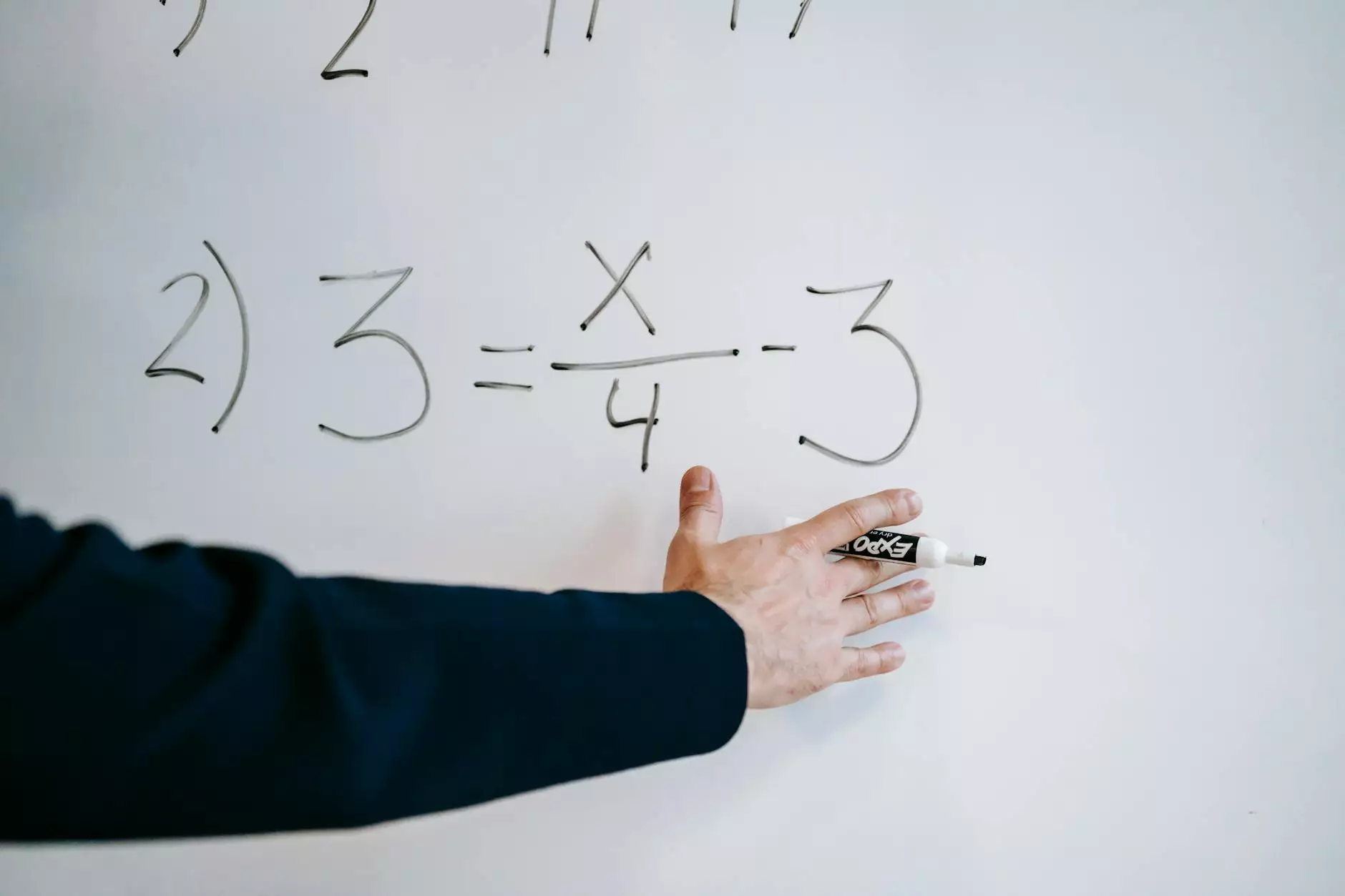
In today's rapidly advancing technological landscape, machine learning has emerged as a cornerstone of innovation across various industries. A crucial element of machine learning is the use of data, particularly visual data. Alongside textual data, videos are an essential type of content that requires meticulous processing. This is where a video annotation tool for machine learning becomes invaluable. This article delves into the world of video annotation and its pivotal role in optimizing machine learning processes.
Understanding Video Annotation
Video annotation refers to the process of labeling or annotating video data. This is a critical step in preparing datasets for machine learning algorithms, allowing machines to understand and learn from visual information effectively. Video annotation can include various techniques, such as:
- Object detection: Identifying and labeling objects within a video.
- Action recognition: Annotating specific actions or movements performed in the video.
- Event tagging: Marking significant events or occurrences within a video sequence.
- Scene segmentation: Dividing the video into meaningful sections for better context understanding.
Why Video Annotation is Essential for Machine Learning
As machine learning strives to emulate human cognition, the quality and accuracy of training data become paramount. Here are some reasons why utilizing a video annotation tool for machine learning is essential:
1. Enhancing Model Accuracy
Annotated videos provide clear, structured data that machine learning models need to learn and improve. By providing detailed labels for various components within a video, these tools ensure that models can differentiate between objects and actions with a high degree of accuracy.
2. Supporting Supervised Learning
In supervised learning, models learn from labeled datasets. Video annotation tools significantly facilitate this by allowing data scientists to create well-organized training sets. The more accurate and detailed the annotations, the better the model can predict and classify outcomes.
3. Enabling Advanced Applications
From self-driving cars to security surveillance systems, the applications of video annotation in machine learning are vast. These tools allow for the development of sophisticated algorithms that can interpret and act on visual data, driving innovations in numerous sectors.
Applications of Video Annotation Tools
Video annotation tools cater to various industries, enabling innovative solutions and enhancing operational efficiency. Some key applications include:
1. Autonomous Vehicles
In autonomous driving, understanding video inputs from sensors and cameras is crucial. Annotated video data allows machine learning models to recognize pedestrians, traffic signals, and obstacles, ensuring safer navigation.
2. Healthcare
Video annotation plays a significant role in healthcare for monitoring patients and analyzing movements. Machine learning models can learn from annotated videos of patients' physical therapy sessions to optimize rehabilitation protocols.
3. Security and Surveillance
In security applications, video annotation is critical for identifying suspicious behaviors or incidents. Annotated footage can train surveillance systems to recognize potential threats and enhance real-time monitoring capabilities.
4. Sports Analytics
Sports teams employ video annotation to analyze player performances. By annotating plays, movements, and strategies, teams can develop tailored training programs that enhance player effectiveness.
Choosing the Right Video Annotation Tool
With numerous video annotation tools available in the market, selecting the right one can be daunting. Here are some key features to consider:
- User-Friendly Interface: The tool should have an intuitive design that allows users to easily navigate through annotations and edits.
- Customization Options: Look for tools that allow you to create personalized annotation templates and workflows suited to your specific needs.
- Integration Capabilities: The tool should integrate seamlessly with existing machine learning frameworks and datasets to streamline the workflow.
- Collaboration Features: For teams working on projects, collaborative features are essential to allow multiple users to annotate and edit video data simultaneously.
Benefits of Using Video Annotation Tools
Utilizing video annotation tools for machine learning comes with several advantages:
1. Time Efficiency
Automated annotation tools can drastically reduce the time spent on the annotation process, enabling quicker turnaround times and faster model training. This is particularly beneficial for businesses seeking to accelerate their development cycles.
2. Enhanced Data Quality
A significant aspect of machine learning is the quality of data used for training. Video annotation tools enhance data quality by providing accurate, detailed labels, which leads to better-performing models.
3. Scalability
As businesses grow, so does the volume of data they handle. Video annotation tools allow for scalable solutions that can accommodate increasing amounts of video data without compromising quality.
4. Cost-Effectiveness
While there may be an upfront investment in acquiring a professional video annotation tool, the cost savings gained from improved model accuracy and reduced time on project deliverables often outweighed the initial expenses.
Case Study: Successful Implementation Example
Consider a tech company focusing on developing advanced surveillance systems. By utilizing a video annotation tool for machine learning, they were able to annotate thousands of hours of video footage, allowing their algorithms to understand various scenarios. As a result, the surveillance system improved its threat detection capabilities, leading to enhanced security provision. This success story highlights the transformative impact of video annotation tools in real-world applications and their capacity to drive innovation.
The Future of Video Annotation in Machine Learning
The realm of video annotation is poised for significant advancement. As technology evolves, we can expect:
- Increased Automation: Machine learning itself will facilitate smarter annotation tools, providing automated solutions that require less manual input.
- Enhanced Accuracy through AI: AI-driven tools will improve annotation precision by learning from previous annotations and correcting mistakes, leading to superior datasets.
- Broader Accessibility: Ongoing developments will make video annotation tools more affordable, accessible to smaller businesses, and diverse industries.
In conclusion, a video annotation tool for machine learning is not merely an accessory; it is a necessity for any organization looking to leverage the power of visual data effectively. By understanding its importance, applications, and benefits, businesses can position themselves at the forefront of technological innovation and prepare for a future driven by data.
Conclusion
As the landscape of machine learning continues to evolve, the role of video annotation tools becomes increasingly significant. Their ability to enhance data quality and streamline processes is invaluable for industries ranging from healthcare to automotive. With an ever-growing demand for precise data and advanced AI solutions, investing in the right video annotation technology is a crucial step for any organization aiming to thrive in the data-driven future.